Publications
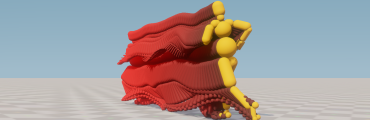
SuperTrack: Motion Tracking for Physically Simulated Characters using Supervised Learning
ACM SIGGRAPH Asia '21
Levi Fussell, Kevin Bergamin, Daniel Holden
Webpage • Paper • Video • Article
In this research we present a method for motion tracking of physically simulated characters which relies on supervised learning rather than reinforcement learning. To achieve this we train a world-model to predict the movements of the physically simulated character and use it as an approximate differentiable simulator through which a policy can be learned directly. Compared to previous methods, our approach is faster to train, has better animation quality, and scales to much larger databases of animation.
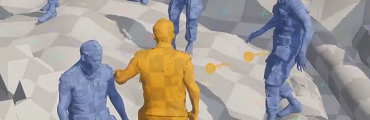
Learned Motion Matching
ACM SIGGRAPH '20
Daniel Holden, Oussama Kanoun, Maksym Perepichka, Tiberiu Popa
Webpage • Paper • Video • Supplementary Video • Article • Code • Data
In this research we present a drop-in replacement to Motion Matching which has vastly lower memory usage and scales to large datasets. By replacing specific parts of the Motion Matching algorithm with learned alternatives we can emulate the behavior of Motion Matching while removing the reliance on animation data in memory. This retains the positive properties of Motion Matching such as control, quality, debuggability, and predictability, while overcoming the main core limitation.
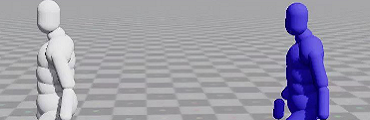
DReCon: Data-Driven responsive Control of Physics-Based Characters
ACM SIGGRAPH Asia '19
Kevin Bergamin, Simon Clavet, Daniel Holden, James Richard Forbes
Paper • Video • Article • Data
In this research we present a method for interactive control of physically simulated characters. Unlike previous methods which either track fixed animation clips or have very unresponsive interactive control, we put an interactive Motion Matching based kinematic controller inside the training environment, controlled by a virtual player, and train the Reinforcement Learning to imitate this controller. This allows for fast and responsive interactive control appropriate for applications such as video games.
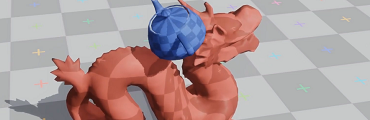
Subspace Neural Physics: Fast Data-Driven Interactive Simulation
ACM SIGGRAPH/Eurographics SCA '19
Daniel Holden, Bang Chi Duong, Sayantan Datta, Derek Nowrouzezahrai
Webpage • Paper • Video • Article • GDC Talk
In this research we show a method of accelerating specific physics simulations using a data-driven approach that combines subspace simulation with a neural network trained to approximate the internal and external forces applied to a given object. Our method can achieve between 300 and 5000 times performance gains on simulations it has been trained on, making it particularly suitable for games and other performance sensitive interactive applications.
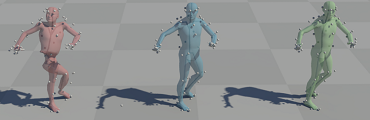
Robust Solving of Optical Motion Capture Data by Denoising
ACM SIGGRAPH '18
Daniel Holden
Webpage • Paper • Video • Article
In this research we present a method for computing the locations of a character's joints from optical motion capture marker data which is extremely robust to errors in the input, completely removing the need for any manual cleaning of the marker data. The core component of our method is a deep neural network which is trained to map from optical markers to joint positions and rotations. To make this neural network robust to errors in the input we train it on synthetic data, produced from a large database of skeletal motion capture data, where the marker locations have been reconstructed and then corrupted with a noise function designed to emulate the real kinds of errors that can appear in a typical optical motion capture setup.
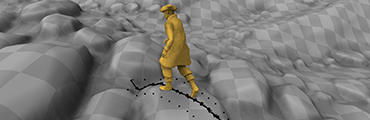
Phase-Functioned Neural Networks for Character Control
ACM SIGGRAPH '17
Daniel Holden, Taku Komura, Jun Saito
Webpage • Paper • Slides • Video • Extras • Demo • Code & Data • Talk • GDC Talk
This paper uses a new kind of neural network called a "Phase-Functioned Neural Network" to produce a character controller for games which generates high quality motion, requires very little memory, is very fast to compute, and can be used in complex and difficult environments such as traversing rough terrain.
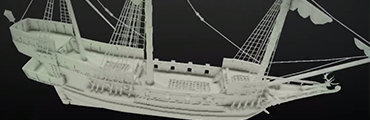
Neural Network Ambient Occlusion
ACM SIGGRAPH Asia '16 Technical Briefs
Daniel Holden, Jun Saito, Taku Komura
Webpage • Paper • Video • Slides • Shader & Filters • Code & Data
This short paper uses Machine Learning to produce ambient occlusion from the screen space depth and normals. A large database of ambient occlusion is rendered offline and a neural network trained to produce ambient occlusion from a small patch of screen space information. This network is then converted into a fast runtime shader that runs in a single pass and can be used as a drop-in replacement to other screen space ambient occlusion techniques.
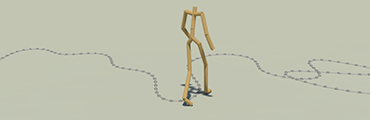
A Deep Learning Framework For Character Motion Synthesis and Editing
ACM SIGGRAPH '16
Daniel Holden, Jun Saito, Taku Komura
Webpage • Paper • Video • Slides • Code • Data • Talk
In this work we show how to apply deep learning techniques to character animation data.
We present a number of applications, including very fast motion synthesis, natural motion editing, and style transfer - and describe the potential for future applications and work. Unlike previous methods our technique requires no manual preprocessing of the data, instead learning as much as possible unsupervised.
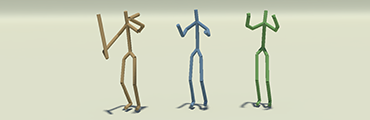
Learning Motion Manifolds with Convolutional Autoencoders
ACM SIGGRAPH Asia '15 Technical Briefs
Daniel Holden, Jun Saito, Taku Komura, Thomas Joyce
Webpage • Paper • Video • Slides
In this work we show how a motion manifold can be constructed using deep convolutional autoencoders.
Once constructed the motion manifold has many uses in animation research and machine learning. It can be used to fix corrupted motion data, fill in missing motion data, and naturally interpolate or take the distance between different motions.
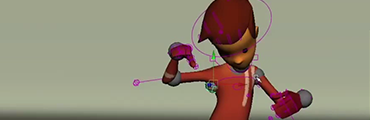
Learning an Inverse Rig Mapping for Character Animation
ACM SIGGRAPH/Eurographics SCA '15
Daniel Holden, Jun Saito, Taku Komura
Webpage • Paper • Video • Slides • Journal Paper
In this work we present a technique for mapping skeletal joint points, such as those found via motion capture onto rig controls, the controls used by animators in keyframed animation environments.
This technique performs the mapping in real-time allowing for the seamless integration of artistic tools that work in the space of the joint positions to be used by key-framing artists - a big step torward the application of many existing animation tools for character animation.
Other Publications
Google Scholar
HUMOS: Human Motion Model Conditioned on Body Shape
European Conference on Computer Vision '24 • Shashank Tripathi, Omid Taheri, Christoph Lassner, Michael Black, Daniel Holden, Carsten Stoll
ZeroEGGS: Zero-shot Example-based Gesture Generation from Speech
Computer Graphics Forum '23 • Saeed Ghorbani, Ylva Ferstl, Daniel Holden, Nikolaus F. Troje, Marc-André Carbonneau
Fast Neural Style Transfer for Motion Data
IEEE Computer Graphics and Applications '17 • Daniel Holden, Ikhsanul Habibie, Taku Komura, Ikuo Kusajima
Carpet unrolling for character control on uneven terrain
ACM SIGGRAPH/Eurographics MIG '15 • Mark Miller, Daniel Holden, Rami Al-Ashqar, Christophe Dubach, Kenny Mitchell, Taku Komura
A Recurrent Variational Autoencoder for Human Motion Synthesis
British Machine Vision Conference '17 • Ikhsanul Habibie, Daniel Holden, Jonathan Schwarz, Joe Yearsley, Taku Komura
Scanning and animating characters dressed in multiple-layer garments
The Visual Computer 2017 • Pengpeng Hu, Taku Komura, Daniel Holden, Yueqi Zhong